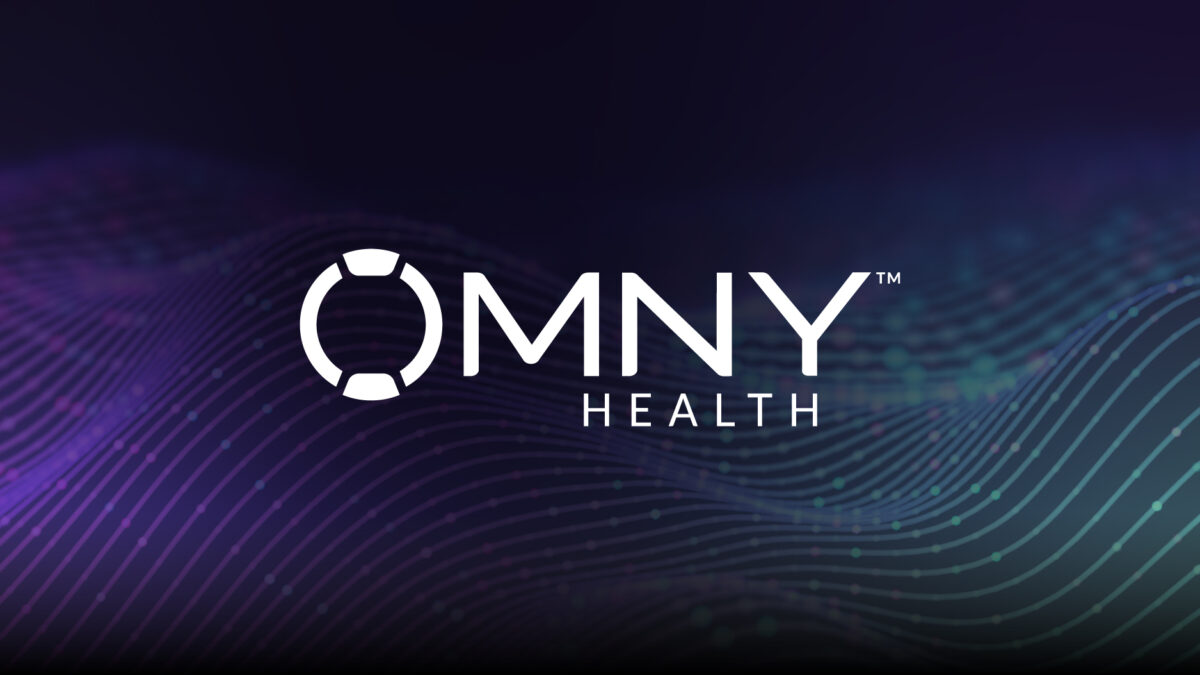
Author: Jona Kerma
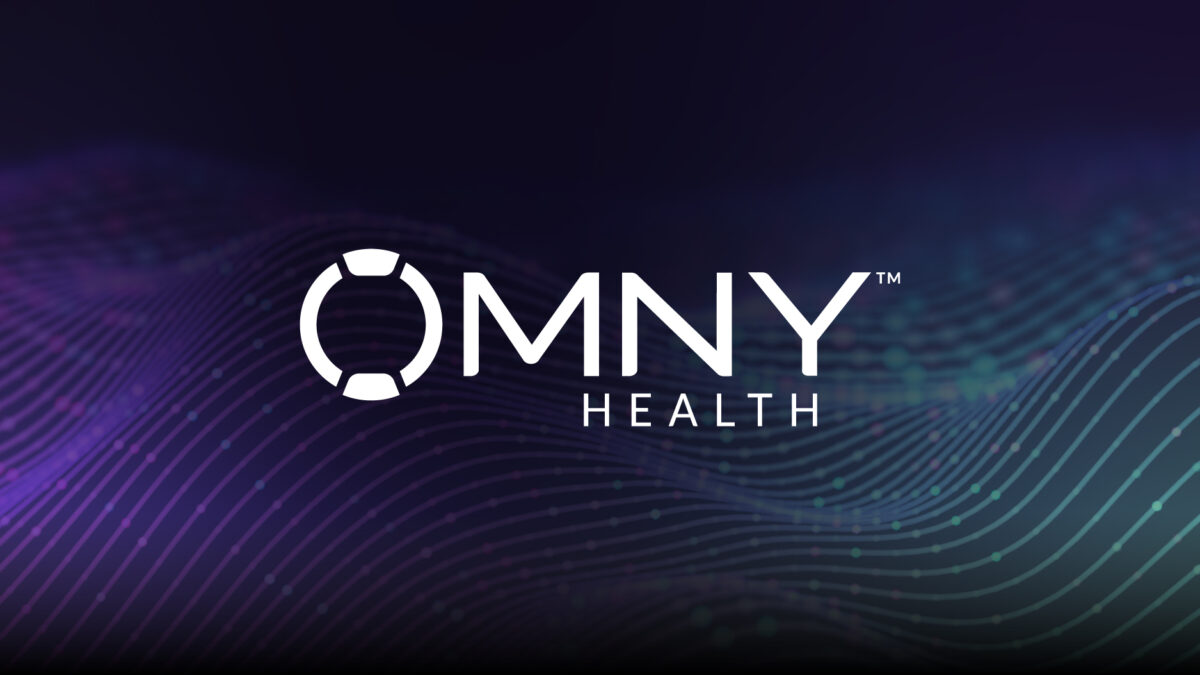
OMNY Health and Scipher Medicine Partner to Revolutionize Autoimmune Disease Treatment with Clinico-Transcriptomics Data
- Post author By Jona Kerma
- Post date January 9, 2025
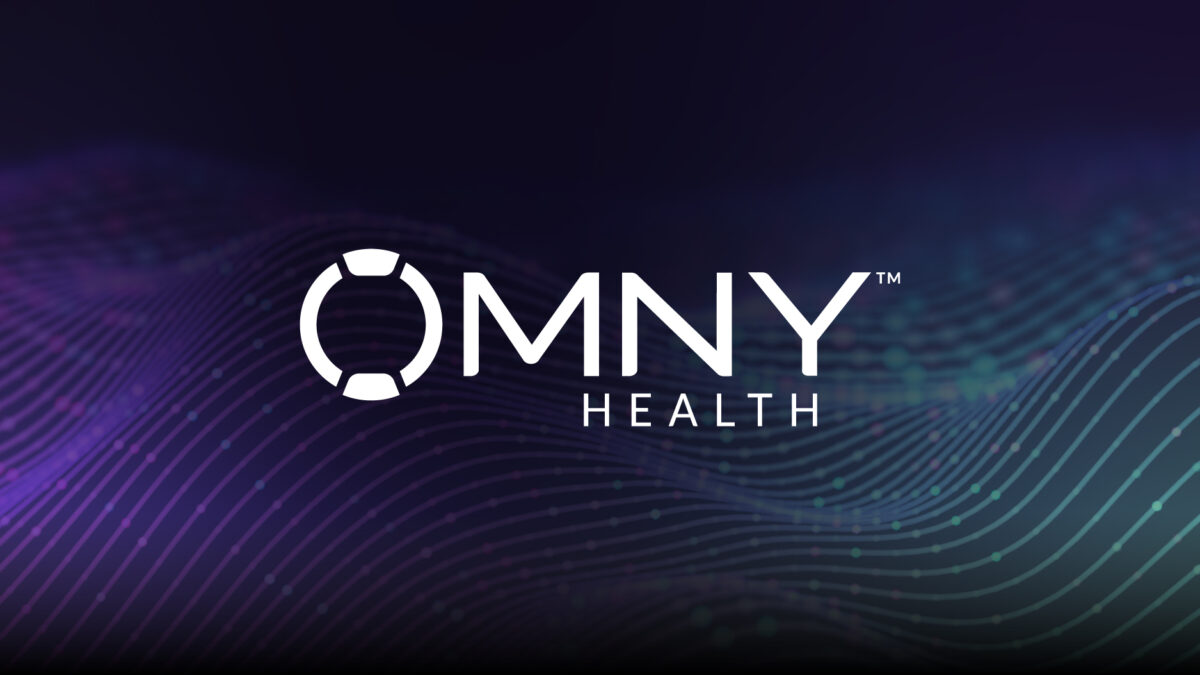
OMNY Health Launches Dynamic GLP-1 Network of 600,000+ Patients to Fuel Clinical Research on Its Use
- Post author By Jona Kerma
- Post date August 27, 2024
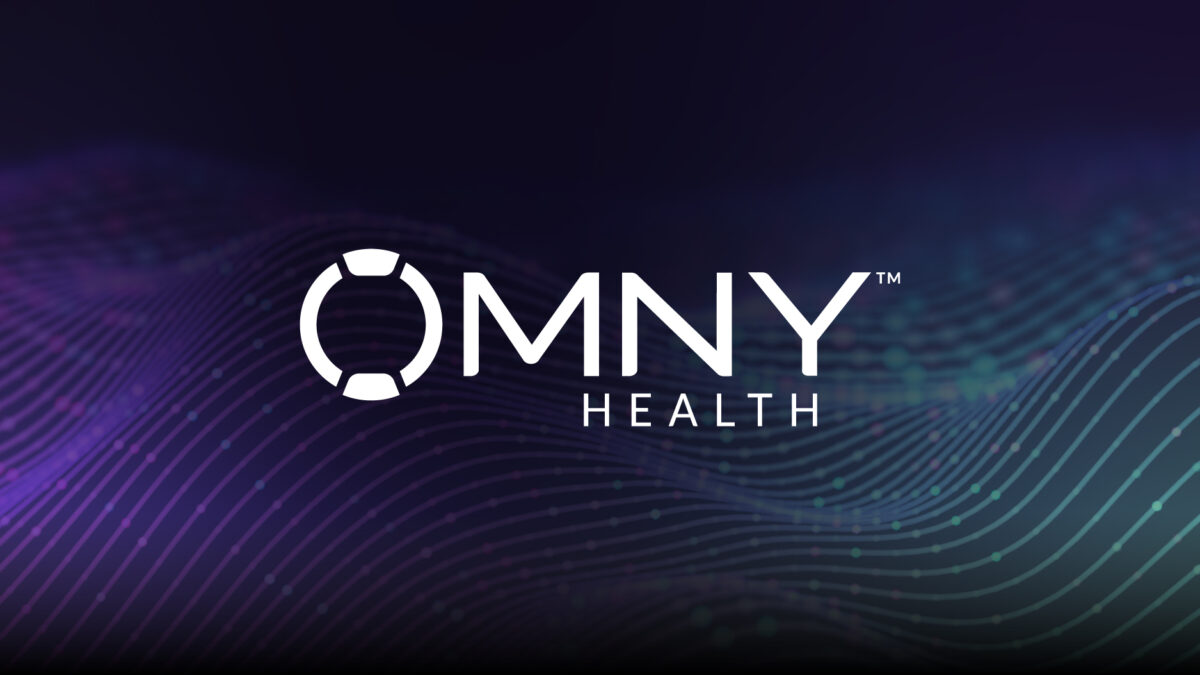
New Studies on the Use of These Medications in Adult and Pediatric Populations are Supported by the Data Network
Atlanta, Georgia – August 27, 2024 – OMNY Health, the leading healthcare ecosystem known for facilitating compliant cross-industry data partnerships, today announced the launch of its Glucagon-Like Peptide-1 (GLP-1) data network, further cementing the company’s commitment to democratizing healthcare data across industries. This new data network comprised already of over half a million patients will supply its life sciences and health system partners, as well as its AI-driven health tech partners, with additional knowledge of GLP-1 use, related social determinants of health (SDOH), and patient demographics to better inform generative AI tools, researchers, and clinicians and improve patient outcomes.
Despite a staggering amount of healthcare data being generated across health systems and life sciences companies, 97% of this data goes unused. This underutilization is particularly crucial as the usage of GLP-1 agonists surge, driven by their effectiveness in treating chronic conditions such as type 2 diabetes and obesity. The growing demand for these medications has sparked an urgent need for more comprehensive data that evaluates their effectiveness and long-term effects for other conditions like cardiovascular disease, liver disease, and skin disorders.
“GLP-1 therapies are transforming the treatment landscape across multiple conditions beyond diabetes and obesity in profound ways. The systemic impact of these medications underlines the need for more comprehensive data to fully understand how these therapies affect patients as a whole,” said Dr. Mitesh Rao, CEO of OMNY Health. “By democratizing data partnerships between life sciences companies and health systems, we can enable more in-depth clinical research that will fuel healthcare innovation and revolutionize the potential impact of GLP-1’s for years to come.”
OMNY’s GLP-1 data network consists of curated EMR data from more than 645,000 patients representing a broad set of demographic characteristics, including age ranges, race/ethnicity, region of care delivery, as well as provider and payer types. The data network supported two recent studies that address gaps in understanding GLP-1 therapies, particularly within pediatric and patient populations impacted by SDOH factors enabling partner companies to better understand and apply findings to patient treatments.
Pediatric use of GLP-1s was not approved by the FDA until December 2022, making data on these use-cases extremely limited despite the prescriptions of GLP-1RAs for children and adolescents increasing by 594.4% from 2020 to 2023. OMNY’s GLP-1 network contains valuable information on pediatric GLP-1 use, enabling a study that found the median age of users was 16 years and the majority were female in gender (72%).
“We are able to deliver more equitable clinical outcomes when the research is backed by data from diverse populations,” said Dr. Sameer Badlani, Fairview Health Services’ Executive Vice President, and Chief Strategy Officer. “With access to data that factors in SDOH for diverse populations we learn valuable insights in service of providing resources and clinical care that is differentiated by excellence in quality, safety, experience and health equity for every consumer who trusts us with their wellness and care.”
OMNY’s network also provides critical information on SDOH factors, including economic insecurity, food insecurity, and social isolation often documented in clinician notes. With many of these factors accounting for up to 50% of the variation in health outcomes in the US, it was crucial that the SDOH status of patients receiving GLP-1 treatment be made available. A second study by OMNY found that GLP-1 patients were half as likely to have SDOH economic burden issues noted in their EHR record as compared the population of non-users.
OMNY’s data network enables companies to diversify their patient populations for clinical trials, better understand medication interactions, and strengthen treatment outcomes.
This announcement follows OMNY’s partnerships with QuantHealth, the leading AI-driven clinical trial design company, and ArisGlobal, a technology company at the forefront of life sciences and the creator of LifeSphere®. OMNY Health is committed to AI-enabled healthcare improvement and supporting research programs that aim to improve clinical care. To learn more about how OMNY Health is transforming lives and driving patient care by connecting providers and life sciences companies through data, visit www.marketing-dev.omnyhealth.com.
About OMNY Health
OMNY Health™ is a national data ecosystem connecting the world of healthcare to fuel partnerships that improve clinical outcomes and drive patient care. OMNY’s dynamic partnerships with specialty health networks, healthcare systems, academic medical centers, and integrated delivery networks span all fifty states and cover over 75 million patient lives. The company’s comprehensive data layer powers health tech companies to drive the next generation of innovation. The platform serves as a centralized resource for life sciences and healthcare provider groups to facilitate mutually-beneficial data sharing and research collaboration at scale, fueling innovation where patients need it the most. OMNY Health’s data ecosystem now reflects more than seven years of historical data encompassing more than 2 billion clinical notes from 300,000+ providers across 200+ specialties – and is growing. For more information, visit www.marketing-dev.omnyhealth.com.
Media Contact:
Chloe Fredericksdorf
omnyhealth@solcomms.co.
OMNY Health Launches Data Platform Designed to Power AI-Driven Health Tech Companies
- Post author By Jona Kerma
- Post date July 2, 2024
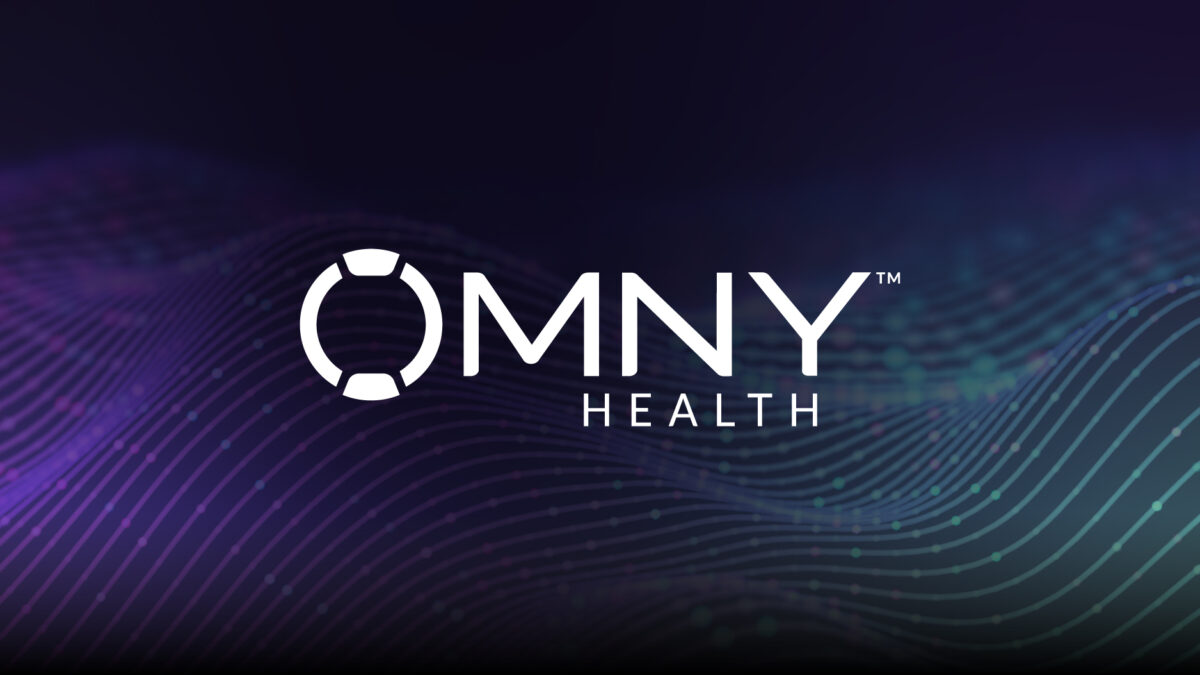
OMNY Health, the leading healthcare ecosystem known for facilitating compliant cross-industry data sharing launched its platform designed to power AI-driven health technology companies, further cementing the company’s commitment to democratizing healthcare data. QuantHealth and ArisGlobal are just two of OMNY’s newest partners to leverage this new platform to power their organizational AI-driven needs. OMNY Health’s data network uniquely addresses Generative AI’s need for large de-identified structured and unstructured electronic health record (EHR) data sets across diverse provider and patient populations, further underscoring the platform as delivering the data infrastructure and rails for provider organizations to collaborate and fuel the development and use of effective AI solutions for advancing healthcare.
“Our mission is to free data from silos, allowing it to be shared, analyzed, and morphed into life-saving treatments and better care for patients,” said Mitesh Rao, CEO, OMNY Health. “Life science organizations and providers often struggle with time and budget constraints that hinder their ability to learn from their data. Our work powering AI-driven platforms can unblock this process by partnering with AI developers with data and tools to accelerate breakthrough therapies and monitor new therapies for safety signals to ensure patient populations remain safe.”
Two of OMNY Health’s AI-driven partners being effectively powered by the platform’s data to foster new opportunities for advancing healthcare include QuantHealth, the leading AI-driven clinical trial design company, and ArisGlobal, a technology company at the forefront of life sciences and creator of LifeSphere®.
QuantHealth’s AI technology, trained on a dataset of 350 million patients, enhances clinical trial timelines, mitigates trial risks, and identifies populations likely to respond to treatments. OMNY’s collaboration will help QuantHealth’s pharmaceutical partners expedite drug development through simulated clinical trials, utilizing real-world evidence to predict clinical trial outcomes, drug efficacy, and patient responses.
“Biotech is entering a vibrant part of life sciences history,” said Omri Matalon, VP Clinical Data Science and Head of R&D Operations at QuantHealth. “This partnership furthers our commitment to quality data and access for our life sciences partners. By integrating diverse EHRs into organized, de-identified research data products, we can address disparities in clinical trials and close significant demographic information gaps in drug discovery and development.”
OMNY’s partnership with ArisGlobal, a leader in pharmacovigilance, safety monitoring, and reporting systems, will transform safety signal validation and pave the way for comprehensive, proactive signal detection.
“Up to 95% of adverse event cases go unreported today, while processing ICSRs can be time consuming, and analysis of medical literature and online forums is slow and inefficient,” said Ann-Marie Orange, CIO and Global Head of R&D at ArisGlobal. “Combining LifeSphere products with comprehensive real-time RWD addresses these challenges head-on. By leveraging advanced technology and insights from extensive RWD, we are reshaping drug discovery and development.”
These partnerships demonstrate OMNY Health’s commitment to AI-enabled healthcare improvement through acceleration of diverse participation in clinical trials and the support of research programs that aim to save lives and improve patient outcomes. To learn more about how OMNY Health is transforming lives and driving patient care by connecting providers and life sciences companies through data, visit https://marketing-dev.omnyhealth.com/.
About OMNY Health
OMNY Health™ is a national data ecosystem connecting the world of healthcare to fuel partnerships that improve clinical outcomes and drive patient care. OMNY’s dynamic partnerships with specialty health networks, healthcare systems, academic medical centers, and integrated delivery networks span all fifty states and cover over 75 million patient lives. The company’s comprehensive data layer powers health tech companies to drive the next generation of innovation. The platform serves as a centralized resource for life sciences and healthcare provider groups to facilitate mutually-beneficial data sharing and research collaboration at scale, fueling innovation where patients need it the most. OMNY Health’s data ecosystem now reflects more than seven years of historical data encompassing more than 2 billion clinical notes from 300,000+ providers across 200+ specialties – and is growing. For more information, visit www.marketing-dev.omnyhealth.com.
About ArisGlobal
ArisGlobal, an innovative life sciences technology company and creator of LifeSphere®, is transforming the way today’s most successful life sciences companies develop breakthroughs and bring new products to market. Headquartered in the United States, ArisGlobal has regional offices in Europe, India, Japan, and China. For more updates, follow ArisGlobal on LinkedIn or visit https://www.arisglobal.com/.
About QuantHealth
90% of drugs fail the clinical stage, representing a direct $45B annual waste to pharma companies. To address this challenge at its core, QuantHealth’s Clinical-Simulator predicts how each patient in a clinical trial will respond to treatment, allowing trial design teams to predict how an entire clinical trial will play out and adapt accordingly. Based on its novel AI engine and a vast dataset of 350m patients and over 700K therapeutics, QuantHealth’s simulator can predict clinical trial results with high accuracy, allowing users to answer mission-critical questions such as trial go/no-go, cohort optimization, drug repurposing, and more. QuantHealth was founded by healthcare experts who led commercial, product, and data science at various leading companies in the US and Israel. QuantHealth is backed by expert Life-Science investors in the US, Europe, and Israel and is supported by an advisory board of physicians and scientists from leading academic institutions. To learn more, visit https://quanthealth.ai/.
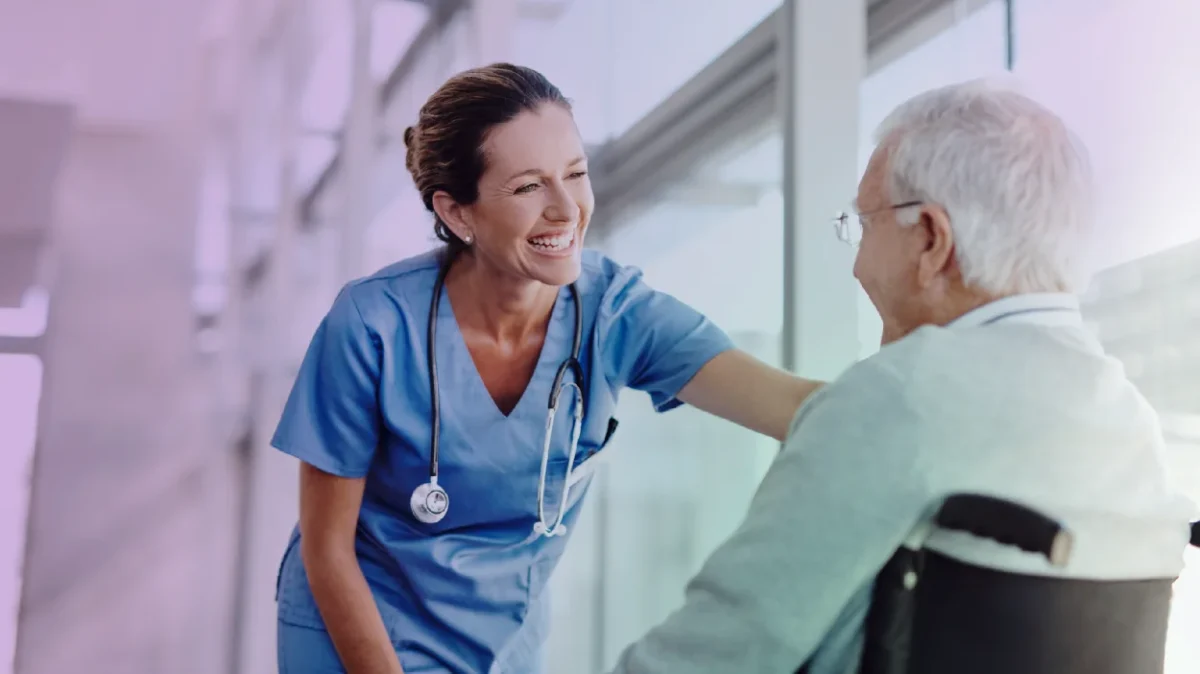
OMNY Health’s GI partnerships are a pivotal step in its mission to deliver data and insights to accelerate life-changing innovation. With a focus on catalyzing research and improving outcomes, OMNY aims to make a tangible difference for the millions of individuals in the US impacted by gastroenterology diseases
ATLANTA, GA, April 22, 2024 – OMNY Health is pleased to announce its partnership with the nation’s leading community-based independent gastroenterology (GI) practices and integrated delivery networks (IDNs) to launch a novel set of real-world data and evidence solutions for research. Over 5,000 GI providers serving more than 10 million patients are now part of OMNY’s research network, representing care delivery by over 380,000 providers and 75 million patients across the US. The focus on GI practice data is a notable expansion to OMNY’s real-world data ecosystem, which works with providers to support compliant research partnerships at scale. OMNY works with the health systems to enable insights on patient population characteristics, care delivery patterns, and treatment outcomes based on data extracted from de-identified inpatient and ambulatory care electronic health records (EHRs). OMNY’s solutions deliver valuable information on disease severity, rationale behind treatment decisions, treatment efficacy, and impact of social determinants on treatment selection and disease progression. By integrating disparate EHRs into unified, de-identified research data products, OMNY offers researchers and providers the breadth and depth of information needed to address their biomedical and population health research questions.
“United Digestive remains committed to offering our patients the most suitable and cost- effective healthcare solutions. Our dedication to delivering best-in-class GI care is intricately linked with our data-driven approach,” says United Digestive’s Chief Medical Officer, Dr. John Suh. “We are thrilled to announce our partnership with OMNY Health, which allows us to drive further insights from our data to advance patient care.”
“As Florida’s leading gastroenterology provider, Borland Groover’s mission is to provide exceptional care and improve the lives of our patients. Achieving this objective requires a deep understanding of our patients, patterns of care, outcomes, and opportunities for further improvement through data analysis,” said Borland Groover’s CEO, Dr. Kyle Etzkorn. “Our collaboration with OMNY not only aligns with our mission, but it also presents avenues to identify and pursue future opportunities for elevating GI patient care.”
“Enhancing patient care isn’t just a goal; it’s our responsibility, guided by the personal experiences of every patient we serve. Each piece of patient data is not just a statistic; it is a pathway to better outcomes, a testament to our commitment, and a source of inspiration for ongoing progress” said Christa Newton, MBA, CEO, OneGI. “We are excited to partner with OMNY as a tangible step in realizing these guiding principles for the benefit of our patients.”
“It is important to One GI that our patients are represented when it comes to emerging research, care improvement initiatives, and best in class care. Our partnership with OMNY helps ensure our providers have the data and support they need to deliver easy to access exceptional GI care.” – Dr. Michael Dragutsky, Founder, Chairman, OneGI. Key populations available in the GI specialty offerings are aligned with the significant research and development efforts underway in gastroenterology, including ulcerative colitis, Crohn’s disease, celiac disease, short bowel syndrome, reflux disorders, and many others. Like other OMNY Health specialty-focused solutions, the GI offering includes information covering pharmacy orders, lab and diagnostic test results, comorbidities, symptom checklists, and provider clinical assessment scores. “We are thrilled to welcome our new gastroenterology providers to our network. Over the past two years, we have made great strides connecting researchers and providers participating in our dermatology and ophthalmology research networks. We look forward to building on that impact in the field of gastroenterology,” said Mitesh Rao, MD, CEO, OMNY Health.
About OMNY Health
OMNY Health connects the healthcare ecosystem through data and insights to transform healthcare delivery, improve clinical outcomes, and address patients’ unmet needs. The OMNY platform serves as a centralized resource for healthcare stakeholders to participate in data sharing and research services at scale, fueling innovation where patients need it the most. For more information, go to www.marketing-dev.omnyhealth.com.
MEDIA INQUIRIES: media@omnyhealth.com
Linking Real-World Clinical Data with Medical Device Data to Demonstrate Device Value
- Post author By Jona Kerma
- Post date April 2, 2024
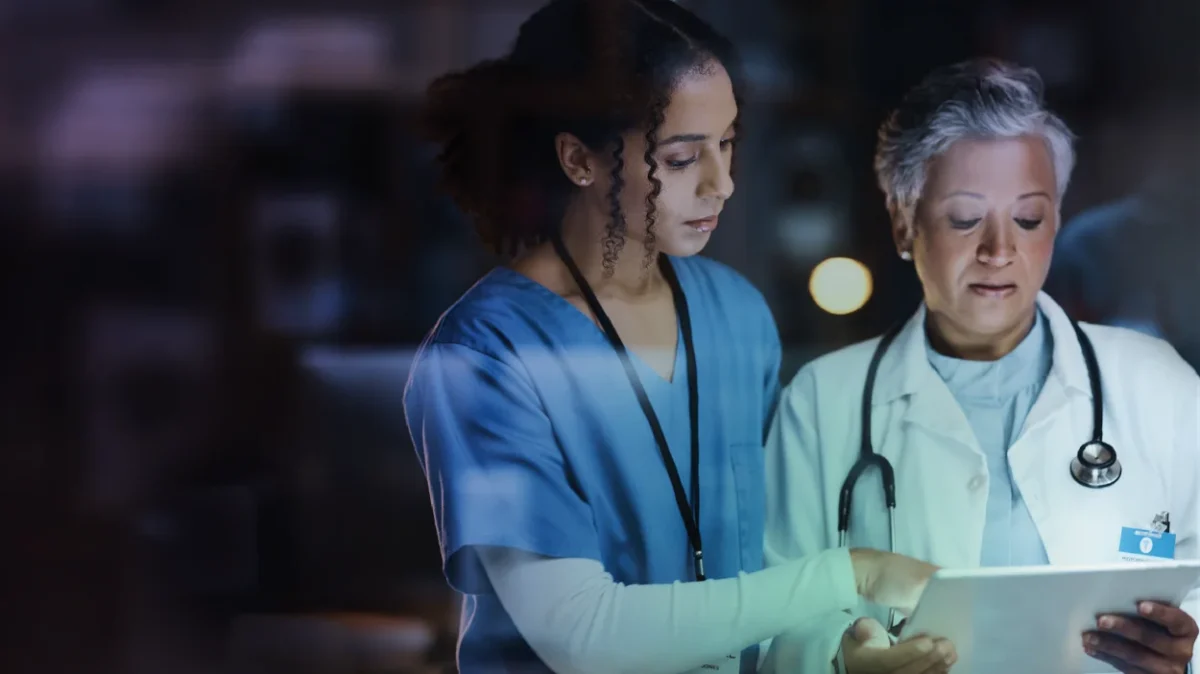
Real-World Data in the Medical Device Market
Many life sciences organizations are focused on harnessing real-world data (RWD) capabilities to inform every part of the drug development product life cycle. The same types of RWD can, and should, be applied to innovation around the development and measurement of the effects of medical devices. RWD derived from healthcare sources, such as electronic health records, provides insights into the burden of disease, and after a device market launch, provides insights into competitive stance, safety, efficacy, and outcomes. Traditionally, these types of analyses have been difficult to perform for medical devices because the specific details surrounding the medical device make, model, and manufacturer used in procedures are often buried in the electronic health record or other IT systems, and historically have not been collected uniformly.
Medical Devices in the Real World
Medical Devices have become a critical part of a patient’s daily life, and how patients interact with these devices can tell us a lot about their effectiveness. From joint replacements to life-saving arterial stents and pacemakers, device manufacturers are interested in the benefit-risk profiles to inform future device modifications and technological advancements, Providers can glean important evidence for the clinical effectiveness of these devices, helping to inform what devices to use in their procedures.
Medical device manufacturers are motivated to invest in new strategies to support success. According to McKinsey, despite a period of growth from 2012 through 2019, S&P medical device investments slowed during the pandemic. Recently, however, signs have been promising; in 2023, the U.S. approved more novel medical technologies than in any single year before, and revenue growth rates are starting to stabilize to pre-pandemic levels.
Aggregation of RWD to Inform Device Safety and Evidence for Effectiveness
Using relevant and reliable RWD is important for generating RWE to understand and regulate medical device usage and safety. To get a complete picture of the patient’s use and interaction with a medical device, RWD from clinically relevant sources is required to give a holistic view of the clinical experience both before device implant/initiation and after usage has been initiated. The ability to combine disparate data from multiple sources and care settings is where OMNY Health excels.
The OMNY Health Medical Device Data Advantage
OMNY Health’s Medical Device product offering is a best-in-class view into the healthcare industry’s use of medical devices in patients. Our Medical Device offering is constructed directly from our health provider data sets, with precision down to the patient encounter level. Utilizing the Unique Device Identifier (UDI) and the device version, we have partnered with the FDA’s AccessGUDID database to ensure the highest quality and completeness of data, with unrivaled recency.
OMNY Health’s Medical Device product offering linked with OMNY Health’s EHR data, can help device manufacturers and provider networks better understand and assist the patients they are servicing by examining:
- Medical Device use and trends
- Patient journeys and continuity of care
- Impact of medical devices on clinical, functional, cost, and utilization outcomes
- Safety/adverse event reporting
- Providers who are interested in value-based contracting and making procurement decisions
Check out the Total Knee Replacement study utilizing the OMNY Health Medical Device product offering.
Contact OMNY to learn more about how our Medical Device product offering will boost your real-world evidence generation.
Social Determinants of Health: Generating Accurate Clinical Insights Using Real-World Data
- Post author By Jona Kerma
- Post date March 6, 2024
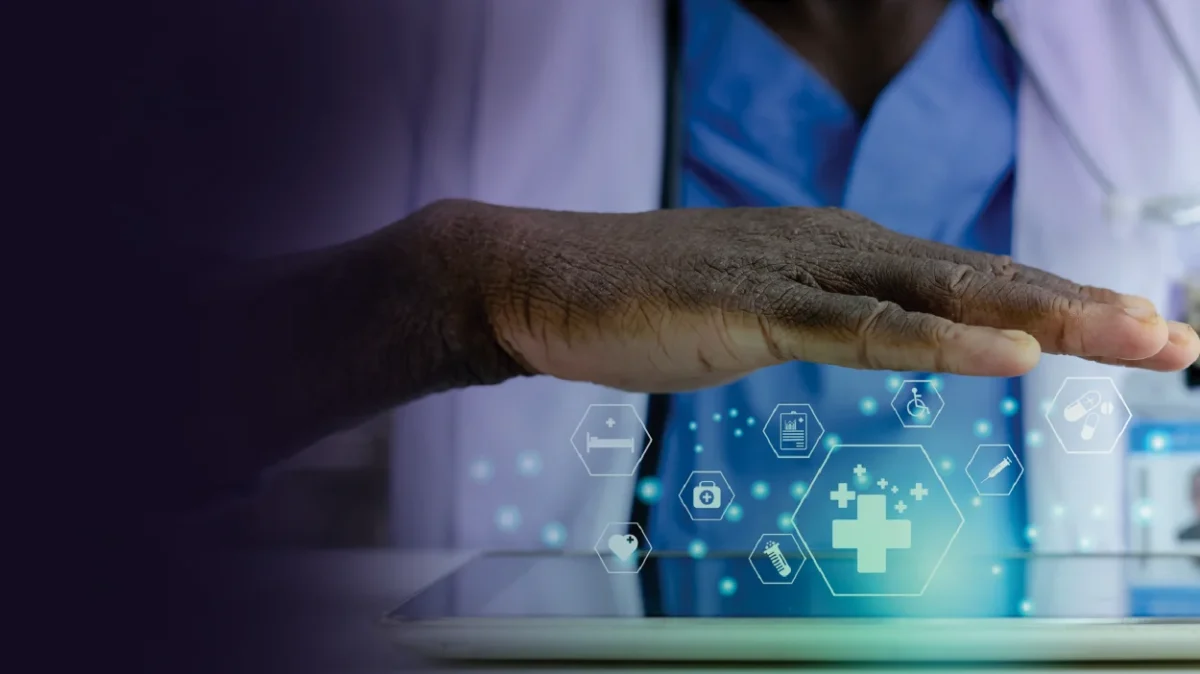
Recent innovations in healthcare analytics and patient-oriented AI demonstrate the power of leveraging real world data (RWD) to generate ecologically valid actionable insights for life sciences companies to improve patient lives and streamline new therapeutic advances. Such insights are essential for decision-making in health economics and outcomes research (HEOR) and in the clinical trial planning and implementation processes. However, the breadth, depth, and inclusivity of the data used to generate such insights can have a huge impact on both the accuracy and validity of any analyses. Various sources of bias, such as the lack of demographic and socioeconomic diversity in a cohort, can undermine the main benefit of using RWD to supplement life sciences research. To combat this, many researchers and providers have publicized the need for clinically-validated metrics that can be used to measure and quantify cohort-level bias and provide more transparency in the field.
Social Determinants of Health Data Account for Notable Variations in Clinical Outcomes
One commonly referenced set of measures that has received growing recognition is social determinants of health (SDoH) data – a set of criteria used to capture economic and social vulnerabilities across key domains. Various studies have estimated that accounting for SDoH variables, such as income level, housing security, transportation, gender identity, and race and ethnicity, can account for 40 – 60% of the variation in clinical outcomes and care patterns. A recent study examining Antihypertensive and Lipid‐Lowering Treatment to Prevent Heart Attack Trial (ALLHAT) data illustrates this all too well. Researchers found that trial participants in the lowest-income trial sites had poorer blood pressure control and worse outcomes for some adverse cardiovascular events, even when treatment access and adherence were accounted for. The authors concluded that these results clearly illustrate “the importance of measuring and addressing socioeconomic context in [randomized controlled trials] RCTs.” The incorporation of these factors into studies leveraging RWD is just as important.
United States Government Support for Clinical Research and RWD Inclusivity
Researchers are not the only ones promoting the importance of SDoH – the U.S. government is facilitating efforts to foster greater inclusivity in clinical trial participation across multiple fronts. The Food and Drug Administration has issued new guidelines about the use of RWD in clinical research, pointing to the utility of inclusive data sources to identify clinical trial participants. The Centers for Medicare & Medicaid Services (CMS) are also focused on health equity. At the end of 2023, CMS released an updated framework for health equity with a focus on the collection of interoperable, standardized SDoH. As of January 2024, CMS requires the screening of five SDOH domains for admitted hospital patients.
Challenges with Obtaining and Utilizing SDoH Data
Despite the power of SDoH to create a more inclusive real-world environment for clinical trials and research analyses, patient-level SDoH data availability to pharmaceutical companies has been lacking. This is in large part due to barriers relating to extracting and aggregating relevant material. In typical healthcare systems, patient-level SDoH data are often difficult to expose and curate as the relevant information is buried in clinical codes and unstructured notes. However, the aforementioned efforts by various government entities have resulted in an exponential increase in the amount of patient-level SDoH data available in medical records – including increased use of structured surveys and other collection tools.
Much of the SDoH data currently available in the RWD marketplace rely on a combination of 3-digit zip-code-level data and aggregated claims or credit data, which only provide inferred modeled proxies, not insight into actual patient-level information. Those that are able to derive SDoH variables through direct access to EHRs often face issues such as data lags, inconsistent data collection vehicles, and gaps in reporting. OMNY Health has the technology in place at the provider level creating a direct flow of data through the OMNY platform so that data is captured consistently with limited lag time. These unique integrated partnerships with large integrated delivery networks (IDNs) and specialty networks have enabled us to quickly capitalize on the growing availability of SDoH data in patient records.
OMNY Health Top-tier SDoH Data Sets
OMNY Health is focused on creating a best-in-class SDoH data product to augment our existing EHR-derived, de-identified clinical data sets. Our Social Determinants data offering is constructed from point-of-care documentation aggregated at the patient-encounter level. To ensure deep, multi-faceted coverage, we leverage multiple streams of information, including ICD-10 diagnostic codes, self-reported responses to standardized SDoH surveys, and estimated SDoH burden derived using research-grade, validated NLP models run on unstructured clinical notes.
OMNY Health’s Social Determinants data offering, when integrated with our extensive clinical data sources, can help pharmaceutical companies and provider networks better help the patients they are serving and be more successful by informing:
- More accurate HEOR analyses
- Inclusive clinical trial planning and implementation
- Analysis of clinical trial results and differences in real-world populations
- The development of precision medicine treatments
- The understanding of the barriers to drug access and adherence and how to develop targeted programs to reduce those barriers
- Different disease severity and outcomes across populations and geographies
- Market access and pull-through strategies for contracting and drug promotion.
SDoH data can offer insight into the everyday non-clinical factors that influence how individuals develop diseases, respond to treatments, and participate in clinical trials.
Contact OMNY to learn more about how our SDoH data sets will boost your real-world evidence generation.
Five Strategies To Boost Clinical Trial Diversity
- Post author By Jona Kerma
- Post date February 28, 2024
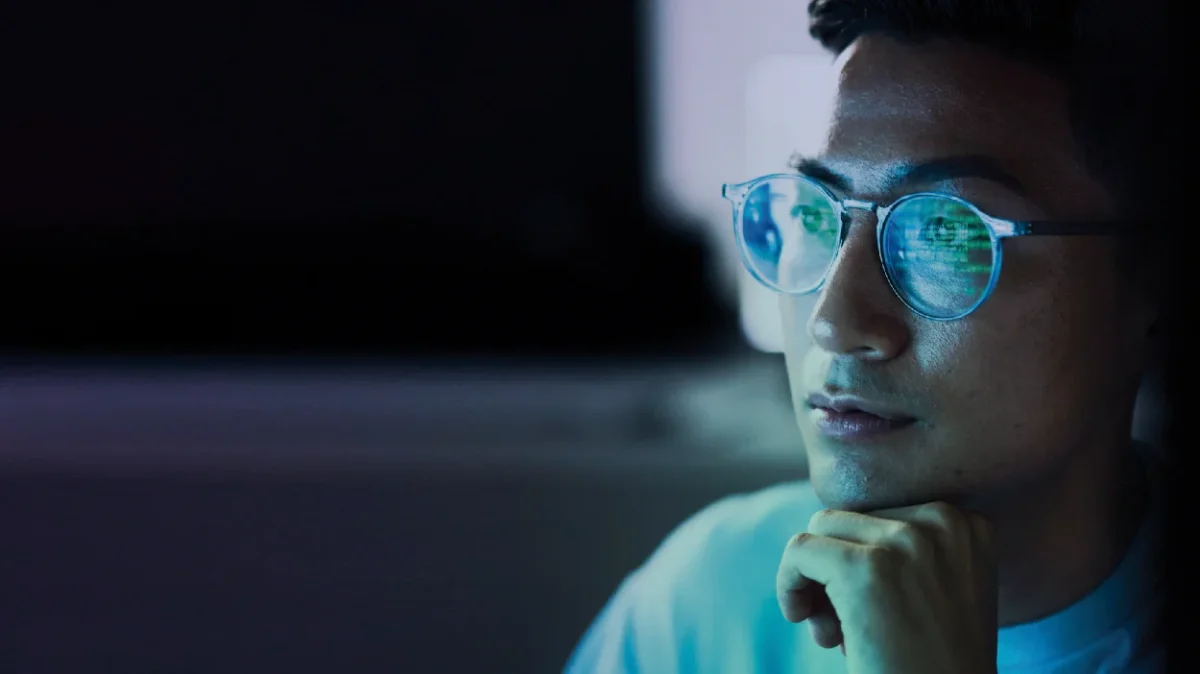
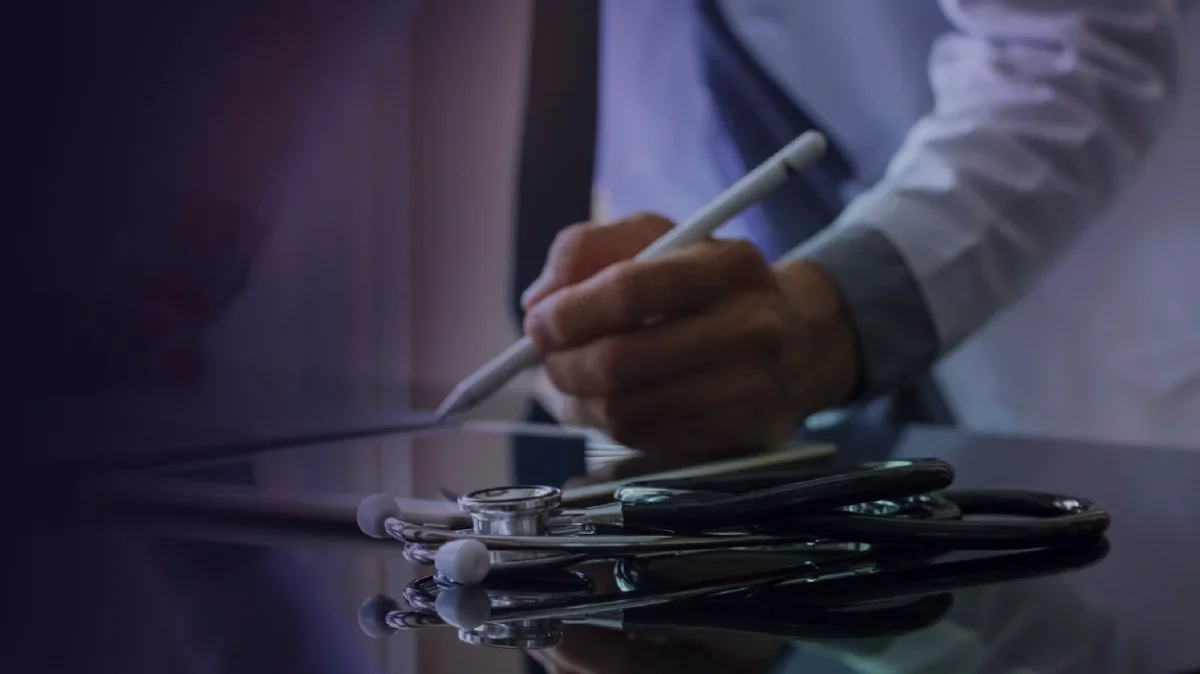
In late 2022, the technology world was turned upside down as OpenAI released ChatGPT, its new artificial intelligence (AI) model. Unlike most previous natural language processing (NLP) models, this model contained billions of parameters, was trained on a corpus of unstructured data with unprecedented size, and underwent a novel alignment process to better orient the model towards human needs. The result was a model that provided superior performance for various NLP-related tasks and professional certification exams (including medical ones) and could produce realistic-sounding text that even the most hardened AI cynics could not deny. Technological and legal impacts were felt almost immediately. Many technology companies began to invest in the new field of generative artificial intelligence (genAI) with an eye toward the potential for financial benefits.
However, lost in all the excitement, is the fact that genAI relies largely on the bread-and-butter NLP methods and subtasks that have supported and improved many industries, including healthcare, over the past decade or two, with the additional capability of text generation. Unlike previous NLP models, newer large language models (LLMs) can perform many different NLP subtasks with one single model.
So, what are the different subtasks that a state-of-the-art LLM can perform? Without further ado, here are five NLP subtasks that can be programmed into LLM to improve healthcare:
Text Classification
Text classification, or the task of distributing text into categories, is often seen as the most primitive NLP subtask. A classic non-healthcare example of this subtask is categorizing reviews (e.g., critiquing products or movies) into having positive or negative sentiments. Text can be classified at the sentence or paragraph level, depending on the use case.
In healthcare, the clinical notes for patients can be classified as to whether they identify patients as having certain attributes or disease characteristics. Using that information, appropriate personalized interventions and treatments can then be used to improve health. Over the past year, work at OMNY Health has focused on several text classification projects, including the following:
- Classifying generalized pustular psoriasis (GPP) patients or patients with a rare, devastating form of the skin disease psoriasis, by their disease status (flare versus non-flare) in collaboration with a life sciences partner.
- Classifying psoriasis patients as to whether they received joint assessments and/or rheumatology referrals in the presence of psoriatic arthritis symptoms, in collaboration with life sciences partners.
- Identifying patients adversely affected by social determinants of health, including illiteracy and financial and housing insecurity.
The results of the study are available (View Report).
Named Entity Recognition
Also known as token classification, named entity recognition (NER) involves classifying individual words or phrases as reflective of an entity, for example, a person, place, or organization. It differs from text classification in that every word is classified, unlike text classification in which sentences and paragraphs are classified.
In healthcare, common entities detected include symptoms, medications, and protected health information (PHI). PHI may be related to individuals, such as names, addresses, and contact information, in larger efforts to deidentify clinical notes before their input in an NLP model. In fact, at OMNY Health, more than 20 PHI entities are identified and removed from clinical text before NLP to protect patient privacy and to comply with the Health Insurance Portability and Accountability Act (HIPAA) of 1996.
Relation Extraction
Relation extraction (RE) represents a relatively more complex subtask in which the relationships between various entities are determined. For example, if two “person” entities are detected in the text, an RE task may be used to identify whether the two entities are married. It is usually performed downstream of an NER step. Previously, RE tasks often required expensive annotation and labeling processes to be performed successfully, although this requirement has faded with the advent of newer LLMs that can label and annotate notes automatically.
In healthcare, RE applications include identifying patient-doctor relationships for clinical note understanding, as well as more general clinical RE to model medical knowledge ontologies such as the Systematic Nomenclature in Medicine – Clinical Terms (SNOMED-CT). At OMNY Health, work has been performed to identify relations between detected symptom and medication entity-pairs to determine if they comprised an adverse drug event (ADE).
Closed-domain Question Answering
Closed-domain question answering is an NLP subtask in which a text is paired with a question about the text, like a reading comprehension question on a standardized exam. The question-context pair is then passed to an NLP model, which then extracts the answer to the question from the text.
In healthcare, question-answering pipelines can be performed to extract practically any conceivable information about a patient from clinical notes, when paired with the correct prompt. The answer can then be processed and refined using additional NLP steps. As an example, a clinical note about medication discontinuation could be passed as input to an NLP model paired with the question, “Why was the medication discontinued?” The answer could then be passed to a text classification model which categorizes the reason for treatment discontinuation. At OMNY Health, we have constructed such a pipeline to determine reasons for treatment discontinuation for various medications (View Report). A second application of question-answering is the extraction of severity scores from clinical notes for various diseases.
Text Generation
Finally, text generation is the process of composing new, original text in response to a provided prompt.
The basic mechanism revolves around predicting the next word based on the input prompt and the sequence of previously generated words. As alluded to in the introduction, modern LLMs undergo alignment to prevent toxic, irrelevant, and/or unhelpful text from being generated. This has resulted in improved output. Text generation can be used for open-domain question answering, in which a general prompt is provided with no corresponding answer in the context (e.g. “What is the purpose of life?”). Text generation is also used on the downstream end of retrieval-augmented generation pipelines, in which a query first causes the NLP pipeline to filter the relevant documents in a database, extract an answer, and then generate text to present the answer to the user.
In healthcare, potential applications of text generation include helping physicians write clinical notes to ease documentation burden, as well as simplifying routine tasks for health analysts at pharmaceutical companies. A true challenge is to accomplish these use cases while protecting patient privacy and preventing any PHI leakage in responses to prompts. At OMNY Health, we strive to accomplish these and related use cases responsibly.
Conclusion
In conclusion, there is more to LLMs than generating text. Combining text generation functionality with the subtasks mentioned in this article potentiates LLMs to complete powerful tasks that can help improve healthcare.
- Tags ai, healthcare
ISPOR Europe 2023: OMNY Health Real-World Data Platform Powering HEOR
- Post author By Jona Kerma
- Post date December 19, 2023
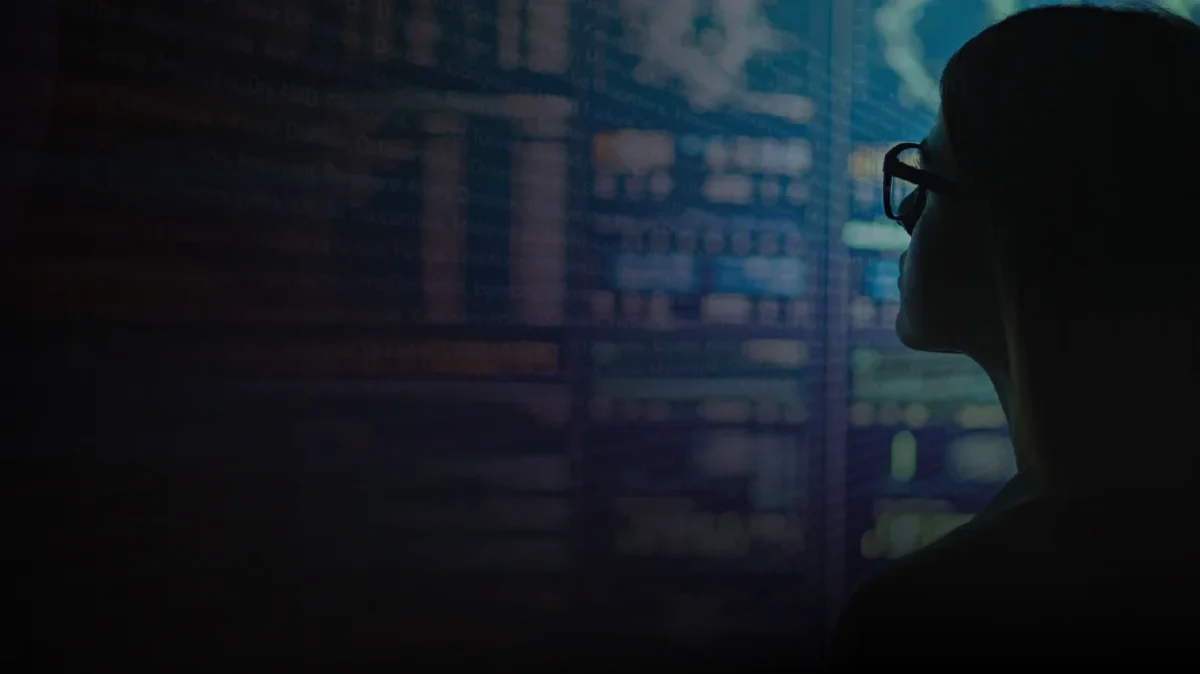
HEOR at the Nexus of Policy and Science
OMNY Health believes in the importance of a truly transparent, secure, and connected real-world data (RWD) ecosystem to accelerate life-changing care and treatment innovation between providers, patients, and life science companies.
Comprehensive, representational de-identified data, standardized and aggregated from the complete EHR record can generate health economics and outcomes research that is truly – like the focus of this year’s ISPOR Europe – at the center of policy and science.
ISPOR Europe was an amazing event this year. There were many common themes among the presentations at the conference, including the harmonization of European data practices and RWD collection and standardization. Other presentations focused on the use of RWD to generate real-world evidence, health technology assessment regulations, and the creation of external clinical trial control arms. Other speakers spoke to the need for RWD-informed policy and science.
HEOR Only as Strong as the Data Behind the Analyses
OMNY Health presented five posters at this year’s ISPOR Europe conference. These posters all focused on using RWD to generate real-world evidence around a variety of topics, including long-term COVID-19, social determinants of health (SDoH), and dermatological diseases. For each poster, OMNY Health scientists utilized the rich EHR data assets in our OMNY Health Platform™ to power new and accurate evidence for the clinical studies presented.
The following is a summary of the purpose and findings of each poster.
1) Long COVID: Clinical Characteristics and Management in the Real-World Setting
A total of 17,542 people with long COVID were studied using OMNY Health data to understand the demographics, concurrent diagnoses, and treatment patterns for those with a long COVID-19 diagnosis code (ICD-10-CM: U09.9). Data were studied from the period of 2021 to June 2023 from four integrated delivery networks and one children’s hospital. Ongoing breathing abnormalities and cough were the most common concurrent diagnoses accounting for 43% of people with long COVID in the study. An analysis of prescription drugs and procedures for these people found that asthma and chronic obstructive pulmonary disease drug treatments were the most common therapies given (25%) with antibacterial agents being the second (13%). The top surgical treatment procedure was radiological at 34%.
This analysis demonstrated how EHR data can be leveraged as a valuable tool to provide insights into chronic disease, clinical characteristics, and disease progression.
2) Identifying economic insecurity among psoriasis patients using natural language processing and unstructured clinical notes
Clinical codes for social determinants of health, such as economic insecurity, exist in the International Classification of Diseases, Tenth Revision, but are often underutilized in clinical practice. This often results in a lack of structured SDoH data recorded in clinical records. However, this type of information can also be found in unstructured data such as clinical notes. The objective of this study was to train and apply a natural language processing model (NLP) to extract economic insecurity information from clinical notes and compare its results identifying economic insecurity from structured clinical coding.
A total of 2,007 clinical note sentences were sampled and annotated to train the model. When the model was applied to almost 51,000 people with psoriasis, 686 people were positive for economic insecurity as opposed to zero people having recorded ICD-10 clinical codes for economic insecurity. Of the 100 positive sentences checked for accuracy, sixty percent were true positives.
This study indicates that NLP can be a useful tool to identify SDoH from the unstructured portions of clinical patient records among patients lacking SDoH ICD-10 codes.
3) Trends in the therapeutic management of bullous pemphigoid in the real-world setting
Bullous pemphigoid (BP) is an autoimmune disorder, often found in the elderly, that causes blisters on the skin. The prevalence of BP is increasing in the US and treatments for BP, including corticosteroids, are associated with toxicities and disease recurrence over time. OMNY Health researchers created a profile of real-world BP treatment patterns across diverse treatment settings in the United States to inform the development of future treatments. They did this by accessing electronic health record data from six multi-state specialty dermatology networks and four integrated delivery networks from 2017 to 2023.
Demographics and disease severity at first BP diagnosis were collected along with the percentages of people with prescriptions for topical corticosteroids (TC), systemic corticosteroids (SC), non-immunosuppressive agents, and immunosuppressants.
- TC prescriptions were the most widely used, increasing from 53% in 2017 to 72% in 2023, peaking at 76% in 2022.
- SC use ranged from 29% to 41%, peaking in 2021.
- Non-immunosuppressive agents increased from 30% in 2017 to 35% in 2023, peaking at 37% in 2022.
- Immunosuppressants were the least used, ranging from 12% to 16%.
These results provided insights into the real-world treatment patterns over time for those with BP. As new treatments are introduced similar analyses could provide insights into uptake and prescription patterns among BP patients at different severity stages.
4) Real-world treatment patterns of ankylosing spondylitis
Ankylosing spondylitis (AS) is an inflammatory disease, affecting the spine, which often spreads to the joints between vertebrae. This disease, which often worsens with age, has treatments that focus on relieving the symptoms of pain and inflammation and preventing longer-term complications. This study examined the real-world treatment patterns of people with AS. These treatments include analgesics and opioids, tumor necrosis factor (TNF) alpha inhibitors, anti-inflammatories, and interleukin 17 (IL-17) inhibitors. Electronic health record data from the OMNY Health RWD platform was accessed for 6,171 people with AS across integrated delivery networks and specialty clinics. Demographic data and the percent of visits with prescriptions were tracked for 5.5 years. Analgesics/opioids were prescribed at 16% of AS-associated patient visits, while TNF alpha inhibitors and IL-17 inhibitors were prescribed at 5% and 1% of AS encounters, respectively.
This study indicates that the long-term electronic health record data found in the OMNY Health RWD platform can be used to provide insights into the therapeutic management of a relatively rare disease such as AS.
5) Itch intensity and therapeutic management of pruritus in the real-world setting
Pruritus, itchy skin, is a cutaneous symptom that presents in a variety of clinical systemic conditions. Its ubiquitous nature is challenging to treat and can greatly impact the lives of those people who have pruritus. Itch intensity scores (1-10) are often documented, especially in dermatological clinics, as a measure of symptom severity. These scores inform pruritus treatment and management.
This study examined the relationship of itch intensity with therapeutic management choices for people with pruritus in six dermatology networks from 2017 to 2013. A total of 7,330 people with pruritus and a recorded 10-point itch intensity assessment associated with a pruritus diagnosis code were studied in the OMNY real-world data platform.
Topical therapies were prescribed at approximately half of pruritus visits, regardless of itch intensity, with topical corticosteroids prescribed at approximately 45% of those visits. Systemic therapy prescriptions were more likely to vary dependent upon itch intensity with 32% of prescriptions associated with the highest itch intensity of 8-10.
This study indicates that the rich dermatological data sets in the OMNY Health RWD platform can be used to provide insights into the management of disease symptoms that greatly impact the quality of life for those who have them.
OMNY Health’s Powerful RWD Platform Informing HEOR and the Impact of SDoH
The 2023 ISPOR Europe conference was an invigorating experience focused on discourse about the changing research landscape in Europe, HEOR, and RWD in the life sciences industry.
OMNY Health values the interactions we had and the opportunities to showcase the strength of our real-world data platform in providing the types of insights that life sciences companies and policymakers seek to understand diseases, treatment patterns, and the impact of disease on the people who have them.